CIOs are increasingly exploiting custom AI stacks and new features in line with business software to automate and streamline business processes — with a few caveats.
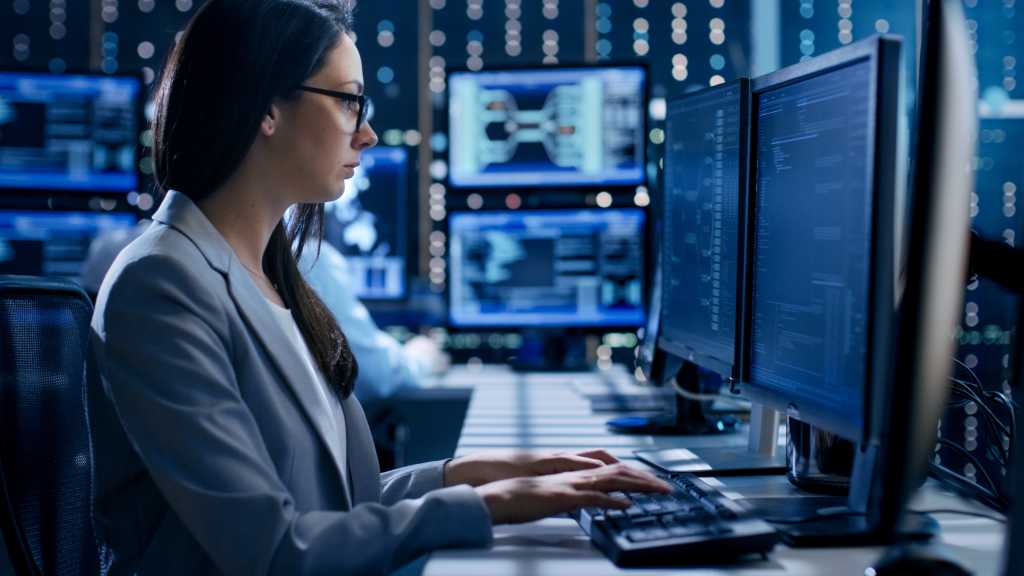
Like other CIOs, Katrina Redmond has been inundated with opportunities to deploy AI that promise to speed business and operations processes, and optimize workflows. “Everyone is running around trying to apply this technology that’s moving so fast, but without business outcomes, there’s no point to it,” says Redmond, CIO at power management systems manufacturer Eaton Corp. “We need to continue to be mindful of business outcomes and apply use cases that make sense.”
Some prospective projects require custom development using large language models (LLMs), but others simply require flipping a switch to turn on new AI capabilities in enterprise software. “AI is showing up in every software package and in every technology, particularly generative AI,” says Dan Diasio, global AI consulting leader at EY, while some vendors, such as Microsoft, have made AI core to their software.
To keep up, Redmond formed a steering committee to identify opportunities based on business objectives, and whittled a long list of prospective projects down to about a dozen that range from inventory and supply chain management to sales forecasting. “We don’t want to just go off to the next shiny object,” she says. “We want to maintain discipline and go deep.”
To be successful, an AI proof of concept (PoC) project also needs to make good business sense, says CIO Vikram Nafde, CIO at Connecticut-based Webster Bank. “The cost of implementing and running AI models can be quite high, so you have to be really careful in assessing the business worthiness of AI use cases,” he says. “This involves rigorous evaluation of potential benefits, risks, and costs associated with each AI initiative to ensure investments are prudent and aligned with our risk-return profile.”
Early positive results
At Eaton, a few PoCs are already generating results and they’ve used AI to consolidate information between more than 70 ERP systems globally. Leveraging expertise at software developer Palantir Technologies, Redmond’s team developed a model that consolidated and cleansed the data from those systems, then analyzed it to provide insights — and fairly sophisticated recommendations — to decision makers.
For example, if production in one business unit is short on eight-inch steel rods needed to finish orders in assembly, and another area of the business has 10-inch rods on hand, the AI might suggest using the longer rods and cutting them down to make the delivery deadline. “A human reviews it to make sure it makes sense, and if it does, the AI incorporates that into the learning model,” she says. The project, which is still in what Redmond calls the “value proposition stage,” has already generated positive results for the company’s electrical business. “On-time delivery has improved substantially,” she says.
Webster Bank is following a similar strategy. “We’ve established an AI working group with representatives across technology, architecture, data, security, legal, risk, and audit consisting of both technical practitioners and business users to develop AI-use best practices and a governance framework,” says Nafde. The bank is also looking at AI to help streamline internal operations and gain efficiencies, including building custom models specifically tailored to the business’ needs. For example, it’s experimenting with using gen AI to automatically read financial statements from corporate customers when assessing loan applications.
“The teams here must read and understand a lot of financial information, and it’s almost never in a standard format,” he says. “Generative AI can read and extract the required information and summarize it for humans.” So far, he says, “We believe it’s a good use case. It can be faster, more accurate, and make the teams more productive.”
Eli Lilly and Company is also at the forefront of adopting and integrating AI into the business. “We’ve found that AI can help in just about every area to streamline workloads and advance our research and development,” says EVP and CIDO Diogo Rau.
Currently, gen AI helps with new pharmaceutical development by creating never-before-seen molecules and analyzing their potential in the development of new medicines. It compresses years of work into months — sometimes days, Rau says. Lilly also developed an AI tool to manage and interpret patient data from therapeutic devices, and improve the safety and effectiveness of medicines using a proprietary “sensor cloud.” Plus, it uses gen AI to automate the development of initial versions of software to produce documentation supporting clinical trials, and to create materials for regulatory submissions.
Production is another area that benefits from AI. “At Lilly sites, we leverage sophisticated algorithms and models, automated guided vehicles, fully automated warehouses, robotics, and highly automated production equipment to increase and accelerate the production of our medicines,” Rau says.
Partnerships are key
To create PoCs for AI projects, CIOs like Redmond at Eaton, are turning to trusted partners for help. “It was essential because we don’t have a plethora of AI resources, and you need a model to start with,” she says. “It’s a good accelerator in the beginning.” But, she adds, it’s also important to move your internal team up the learning curve to keep costs down as projects are put into production.
Webster Bank is leveraging hyperscalers such as Microsoft and AWS whenever possible, and equipping the bank’s own expert technologists to build what’s most important for its needs, all while minimizing reliance on consultants. “That way, we don’t have to spend on expensive contractors for both build and ongoing support,” says Nafde.
Trust, but verify
A successful PoC doesn’t guarantee success: Stakeholders need to trust it. At Eaton, for example, an AI-based sales forecasting tool has the potential to boost productivity dramatically. Currently it takes months and thousands of man-hours for all its finance and sales teams to review historical information, combine it with new sales forecasting data, and create projections. Now, says Redmond, “The AI model can potentially do that for you.”
Eaton’s forecasting PoC project, which ran in Q4 last year, has been at least as accurate, she adds, if not more so than the current approach. “It’s certainly better than what we’re doing now, spending thousands of hours working on it,” she says. The question is whether people will be willing to trust the technology enough to give up doing the work themselves. “We’re not there yet on folks being comfortable letting it go,” she explains. “We’re still in the ‘trust but verify’ phase.”
Another reason why trust in AI might create a trust issue — both inside and outside of IT — is the fact the model is a black box in terms of understanding exactly how the output was determined. “And for the first time, with generative AI, we’re working with technology that’s not deterministic; it’s not binary,” says Sanjay Srivastava, chief digital strategist at Genpact. “For example, with generative AI, you can get an answer that says 94% of the time it’s right, which means it needs some oversight or augmentation.”
“These tools are incredibly powerful and sometimes convincingly wrong,” says EY’s Diasio. Unfortunately, though, there’s a tendency for people to go on autopilot. Humans need to work with the tools and review the output, not just casually, but in detail. “You need to plan the time for that,” he says.
Srivastava says most projects keep a human in the loop to make final decisions, but follow through is key. “How do you go from data, to insights, to action in a continuous loop?” he asks. “That’s the number-one reason why people don’t get economic outcomes.”
Data prep matters, except…
In areas such as supply chain and analytics, having all of your data in a form readily available to an AI model is essential. “Data is the lynchpin to AI success,” says Nafde. “Start with your data strategy before your AI strategy, and align your AI strategy with your business strategy.”
Diasio agrees. “Make sure the data you have is discoverable by AI systems, which might mean building an enriched catalog using generative AI or using it to build an ontology on top of structured data,” he says. “In many instances, it’s a significant improvement in productivity when using AI to streamline these workloads. In some data migration activity we’ve observed a 40% increase in various steps along the way and an increase in speed.”
Lilly is already using AI-enabled tools to speed the ingestion and cleaning of the data used to train and fine-tune its pharma models, Rau says, and Genpact also uses AI to prepare its data for consumption by its AI models. “We have a ton of data and two-thirds of it is unstructured,” says Srivastava. “You can use generative AI to auto build a semantic layer on top of your data. You need to understand what data sits where, how it’s linked to something else, what the quality is, the lineage, and where else it’s being used.”
That work is difficult and requires highly skilled talent, which is why many enterprises bring in a partner to help with the work. But AI can automate the creation of that semantic layer for you. It’s not perfect, but it might get you to 80%, Srivastava says.
However, Diasio says you don’t always need to organize internal data to leverage AI. “For example, with generative AI and the pre-trained models available on the market, creative tasks like product development, or summarization tasks such as contact center transcripts, may work effectively out-of-the-box in the appropriate contextual setting and with clever prompting,” he says. “This can help companies accelerate the use of AI while they continue to curate their internal data and harvest their expertise.”
Ensure suitability of AI capabilities before turning them on
“CIOs should invest in new or upgrade existing CRM, IoT, ITSM and business intelligence tools that include AI/ML,” says Jevin Jensen, research VP at IDC. “Time to value is dramatically reduced when you select a solution from an existing off-the-shelf vendor that has added AI features to software you’ve already implemented.” You may simply need to turn on the feature or add a plug-in. Just check to make sure you can opt out of having your data used to train the vendor’s models, he says.
While new AI capabilities in enterprise software such as those offered by Salesforce and ServiceNow promise substantial workflow productivity benefits, you shouldn’t just turn them on without fully understanding how they fit with your workflows. “We recently had a deep-dive session with ServiceNow on how to use intelligent prediction, virtual chat, and other capabilities in alignment with our business strategy,” Nafde says. For example, the bank’s virtual chat function includes a few dozen use cases. Some may be able to use it right out of the box, some will require customization, and some won’t be fit for purpose. “We need to decide which capabilities will be useful,” he says.
Eaton has already turned on some AI features in ServiceNow, with encouraging results so far. “It’s helping from a case management perspective, finding threads of defects we can improve, finding the root cause, and offering solutions that can reduce case counts,” Redmond says.
The conundrum with embedded AI in enterprise software, though, is it may not offer a compelling solution today for your organization’s needs. In this case CIOs, especially if they face competitive pressures, may find themselves in a dilemma: “Should you wait for your line of business application vendors to incorporate AI and sacrifice time to market while you wait for the vendor to build it, or should you build an enterprise architecture strategy where you have your own custom implementation and infrastructure around it, but it’s expensive and needs ongoing investment?” asks Srivastava. “Therein lies the challenge.”
Lilly is also leveraging AIOps capabilities in its IT operations. AI-enabled tools include an incident detection and response system that swiftly detects anomalies, predicts potential problems before they can escalate, determines root cause of failures, and assesses the business impact of technical issues. “For example, if the order processing system experiences delays, AIOps can quantify the impact on revenue and customer satisfaction,” says Rau. This enables the team to prioritize and resolve the most critical issue faster.
What to do – and not to do
While Webster Bank is still in the early phases of its AI journey, Nafde has learned a few things along the way so far: Get your data in order. Align your AI strategy with your business strategy. Put the right KPIs in place before you start. Then start small, show proof of value, scale gradually, and educate and communicate with your stakeholders every step of the way, he says.
Equally important is to partner to get off the ground, but build out your team with the tools and expertise to develop and maintain new AI capabilities. And don’t underestimate the need to build trust. “Stay ahead on your messaging,” he says. “Expect skeptics, do town halls, and have leaders step in.” After all, there’s a lot of fear and general reluctance to accept change when new technology is introduced. “The challenge here isn’t just about AI,” he adds. “It’s a classic change management problem.”
Be strategic and limit the number of projects you take on, adds Redmond. “Focus on a few things and go deep,” she says. Find trusted partners to help you get started, and take advantage of AI capabilities your SaaS vendors have introduced into their products — when they make sense. Don’t overlook what’s already in your ecosystem, she adds.
“Culture matters,” adds Rau. “Change is tough, so CIOs need to lead a cultural shift by demonstrating the innovative open-minded behaviors you’re looking for, and creating an environment that encourages learning and innovation around AI. Our biggest risk is if our employees don’t use AI as much as they could.”
Getting some wins under your belt, like stakeholders using it until they’re comfortable with the new technology, is a real confidence boost, says Redmond. “That gets the fear factor down,” she says.