Deep Learning for Drone Detection: Enhancing Security and Precision
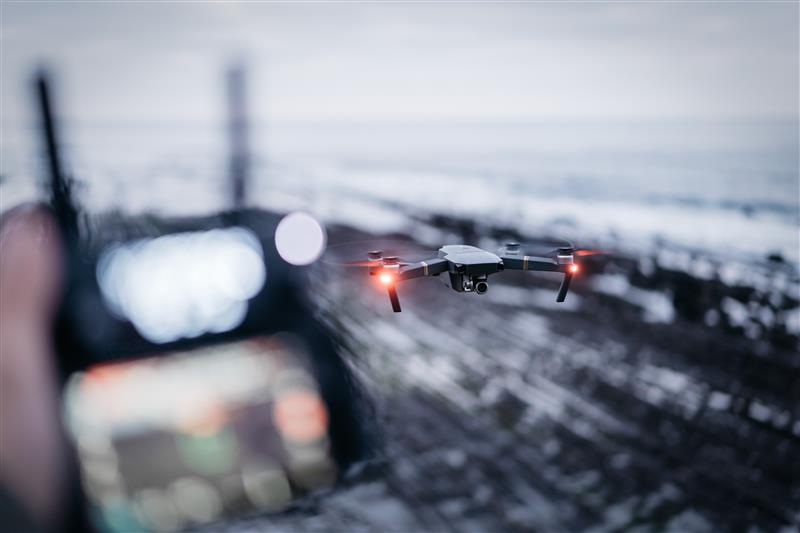
Deep learning for drone detection is used to recognize drone behaviour, detect possible threats, and analyze and categorize drones based on their unique features. These factors may include their form and speed, flying pattern, and trajectory.
Sparked by innovations in processing power, deep learning and capabilities, the world of drone detection has seen great leaps in recent years.
Now, deep learning algorithms are powerful tools capable of supporting operators, assisting in unmanned traffic management, and unlocking pathways for more efficiency and productivity.
Read on to learn more about how deep learning processes are supporting drone detection and perimeter security, and what ongoing evolutions mean for operator workloads and capabilities.
What is deep learning in drone detection?
At its core, deep learning drone detection is a complex branch of Artificial Intelligence (AI) that involves using neural networks to learn from data, draw insights, and even determine an optimum solution. Trained on a set of data, ‘deep learning’ refers to the fact that algorithms have more than one hidden layer of functionality that exists between the input and output stages.
When correctly applied, these algorithms can empower a range of use cases – including providing personalised recommendations and recognising specific images and objects. Within the world of detecting drones, experts are focusing on the best ways to take advantage of deep learning. Just some of the currently identified applications include:
- Accurately detecting and identifying security risks
- Assisting in Unmanned Traffic Management (UTM) operations
- Reducing operator workload
Accurately detecting and identifying security risks
Deep learning can be used for leveraging powerful machine learning models to recognise drones’ behaviour to identify potential risks and analyse—as well as classify—drones based on their specific characteristics. These parameters might include their shape and speed, flight pattern, and trajectory.
For transport hubs such as Heathrow Airport, behavioural analysis is used to identify the difference between bird movement, regular flights and logistics, and a drone flying with intent and speed towards its perimeters.
As there is a distinctive difference in the behaviour between these examples, deep learning-assisted tools can identify risks accordingly, and alert responders to their presence.
Assisting in Unmanned Traffic Management (UTM) operations
Deep learning in detect drones is also being used to assist unmanned traffic management operations, supporting UAS systems operating both within visual line of sight, and beyond visual line of sight (BVLOS). In regulated airspaces, where managing multiple UAVs, where UAV detection is essential, deep learning enhances the accuracy and efficiency of these operations.
On the other hand, Unmanned Traffic Management (UTM) provides certain key functions thanks to its advanced object detection models and sensor fusion. Such pivotal functions include:
- Tracking the deviation of drones
- Identifying drone trajectory and other behaviour outliers
- Sending automated notifications to remote pilots
- Providing guidance in the event of an emergency landing
On top of these scenarios, deep learning applications are supporting current security operators to secure perimeters.
UTM is strongly related to deep learning, especially in detecting drones and ensuring their compliance with airspace regulations. Using deep neural networks, trained on extensive datasets, will improve the recognition and classification of both authorised and unauthorised drones.
This way the system can easily differentiate between different types of aerial objects and issue appropriate alerts thanks to radio frequency signals, drone imagery, and drone sound patterns.
How does deep learning benefit CUAS operators?
With the continued advancements in AI and deep learning technology, we can expect to see even more sophisticated and accurate AI image analytics in the future. As well as providing unique capabilities, deep learning algorithms are supporting operators, boosting efficiency and productivity in the process.
This technological advancement is crucial in keeping pace with the fast development and deployment of drone technology across industries. Now, let’s see how deep learning tools improve the capabilities of operators in drone detection:
- Improved detection accuracy: Deep learning algorithms can analyze large amounts of data from different sensors, including cameras and radar, to accurately identify drones. Such technology can recognize patterns and anomalies, reducing false positives and improving the detection systems’ reliability.
- Real-time processing: Models that have deep learning tech are capable of processing data in real time. This is essential for drone detection systems where timely response is critical.
- Enviromental adaptability and scalability: Deep learning models are trained to perform well in different places and under different conditions. Whether it’s an urban landscape or at large events, the systems can detect drones with ease. The system can scale to handle larger areas and complex scenarios without a significant increase in operational complexity.
- Automated threat assessment: This type of learning can help in assessing the level of threat posed by a detected drone by analyzing its type, payload, flight path, and other parameters.
Minimising required operator inputs
In the past, operators focused on monitoring secure perimeters were required to manually analyse images and video footage, which was a notoriously time-consuming, labour-intensive, and costly task. What’s more, this task needed to be completed 24 hours a day, 7 days a week for complete security, with the capacity to identify and analyse one waveform at a time.
However, with advanced behavioural and image analysis tools now available, sites can minimise the amount of operator input required, enabling them to perform other tasks simultaneously without sacrificing the integrity of their security solutions.
What’s more, these algorithms can analyse hundreds of waveforms simultaneously, offering an exhaustive, reliable and fast overview.
When behavioural and image analysis tools do recognise a risk, operators can quickly verify and coordinate responses, taking advantage of the latest situational intelligence to quickly close the window of vulnerability.
Learn more: why is situational intelligence the key to creating safer spaces?
Reducing the risk of false alarms
Another way that AI image analytics reduces operator workload is by minimising the number of false alarms.
As operators have traditionally been required to operate monitoring devices manually, human error – among other factors – has resulted in false alarms disrupting ongoing regular operations.
However, with deep study and learning-supported analytics bringing an additional layer of security and analysis, systems can be trained with unmatched surveillance to accurately recognise and classify a wide range of objects. This not only reduces disruption but makes operations more efficient than ever in the process.
Developing innovative tools to protect people, property, and planet
At OSL, we are dedicated to creating world-leading solutions that create safer spaces. Leading advanced research into BVLOS operations in complex environments, we provide the expertise, experience, and knowledge needed to seamlessly mitigate risk.
These tools support current security operators by providing better perimeter identification and automating threat detection. The integration of these tools ensures effective drone management, reducing the risk of interference and improving overall airspace safety, especially during complex BVLOS missions.
Learn more by visiting our full range of leading drone detection news, resources, and insights.