Analysis of Algorithms | Big-Omega Ω Notation
Last Updated :
29 Mar, 2024
In the analysis of algorithms, asymptotic notations are used to evaluate the performance of an algorithm, in its best cases and worst cases. This article will discuss Big-Omega Notation represented by a Greek letter (Ω).
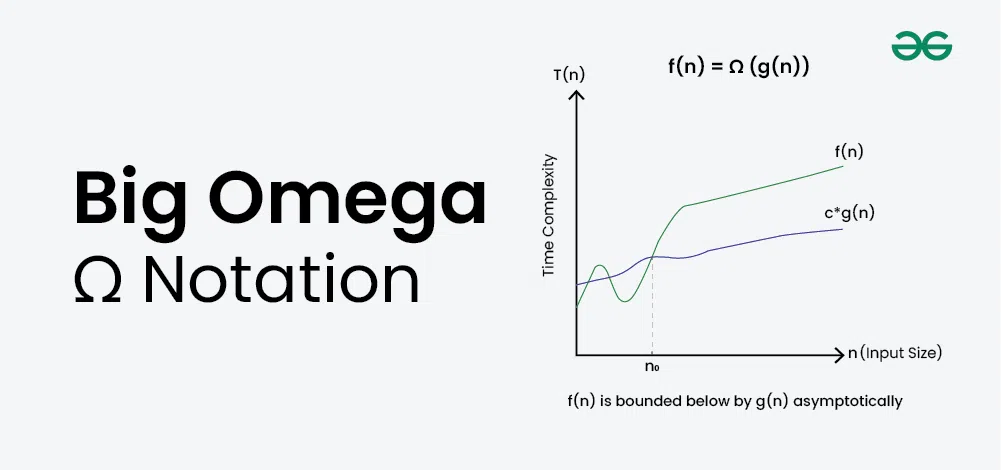
What is Big-Omega Ω Notation?
Big-Omega Ω Notation, is a way to express the asymptotic lower bound of an algorithm’s time complexity, since it analyses the best-case situation of algorithm. It provides a lower limit on the time taken by an algorithm in terms of the size of the input. It’s denoted as Ω(f(n)), where f(n) is a function that represents the number of operations (steps) that an algorithm performs to solve a problem of size n.
Big-Omega Ω Notation is used when we need to find the asymptotic lower bound of a function. In other words, we use Big-Omega Ω when we want to represent that the algorithm will take at least a certain amount of time or space.
Definition of Big-Omega Ω Notation?
Given two functions g(n) and f(n), we say that f(n) = Ω(g(n)), if there exists constants c > 0 and n0 >= 0 such that f(n) >= c*g(n) for all n >= n0.
In simpler terms, f(n) is Ω(g(n)) if f(n) will always grow faster than c*g(n) for all n >= n0 where c and n0 are constants.
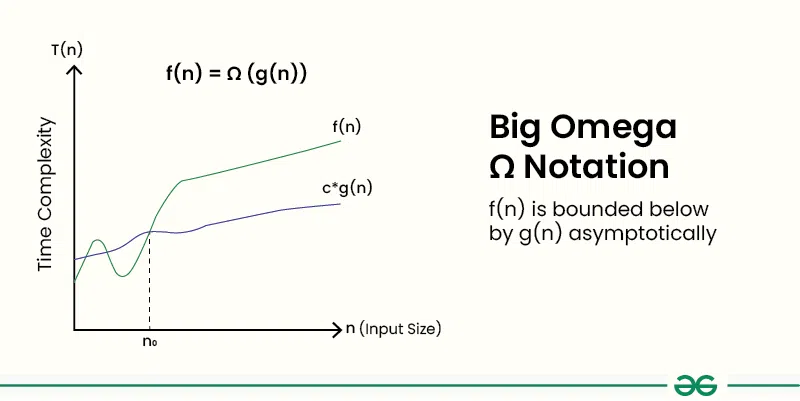
How to Determine Big-Omega Ω Notation?
In simple language, Big-Omega Ω notation specifies the asymptotic lower bound for a function f(n). It bounds the growth of the function from below as the input grows infinitely large.
Steps to Determine Big-Omega Ω Notation:
1. Break the program into smaller segments:
- Break the algorithm into smaller segments such that each segment has a certain runtime complexity.
2. Find the complexity of each segment:
- Find the number of operations performed for each segment(in terms of the input size) assuming the given input is such that the program takes the least amount of time.
3. Add the complexity of all segments:
- Add up all the operations and simplify it, let’s say it is f(n).
4. Remove all the constants:
- Remove all the constants and choose the term having the least order or any other function which is always less than f(n) when n tends to infinity.
- Let’s say the least order function is g(n) then, Big-Omega (Ω) of f(n) is Ω(g(n)).
Example of Big-Omega Ω Notation:
Consider an example to print all the possible pairs of an array. The idea is to run two nested loops to generate all the possible pairs of the given array:
C++
// C++ program for the above approach
#include <bits/stdc++.h>
using namespace std;
// Function to print all possible pairs
int print(int a[], int n)
{
for (int i = 0; i < n; i++) {
for (int j = 0; j < n; j++) {
if (i != j)
cout << a[i] << " " << a[j] << "\n";
}
}
}
// Driver Code
int main()
{
// Given array
int a[] = { 1, 2, 3 };
// Store the size of the array
int n = sizeof(a) / sizeof(a[0]);
// Function Call
print(a, n);
return 0;
}
Java
// Java program for the above approach
import java.lang.*;
import java.util.*;
class GFG{
// Function to print all possible pairs
static void print(int a[], int n)
{
for(int i = 0; i < n; i++)
{
for(int j = 0; j < n; j++)
{
if (i != j)
System.out.println(a[i] + " " + a[j]);
}
}
}
// Driver code
public static void main(String[] args)
{
// Given array
int a[] = { 1, 2, 3 };
// Store the size of the array
int n = a.length;
// Function Call
print(a, n);
}
}
// This code is contributed by avijitmondal1998
C#
// C# program for above approach
using System;
class GFG{
// Function to print all possible pairs
static void print(int[] a, int n)
{
for(int i = 0; i < n; i++)
{
for(int j = 0; j < n; j++)
{
if (i != j)
Console.WriteLine(a[i] + " " + a[j]);
}
}
}
// Driver Code
static void Main()
{
// Given array
int[] a = { 1, 2, 3 };
// Store the size of the array
int n = a.Length;
// Function Call
print(a, n);
}
}
// This code is contributed by sanjoy_62.
JavaScript
<script>
// JavaScript program for the above approach
// Function to print all possible pairs
function print(a, n)
{
for(let i = 0; i < n; i++)
{
for(let j = 0; j < n; j++)
{
if (i != j)
document.write(a[i] + " " +
a[j] + "<br>");
}
}
}
// Driver Code
// Given array
let a = [ 1, 2, 3 ];
// Store the size of the array
let n = a.length;
// Function Call
print(a, n);
// This code is contributed by code_hunt
</script>
Python3
# Python3 program for the above approach
# Function to print all possible pairs
def printt(a, n) :
for i in range(n) :
for j in range(n) :
if (i != j) :
print(a[i], "", a[j])
# Driver Code
# Given array
a = [ 1, 2, 3 ]
# Store the size of the array
n = len(a)
# Function Call
printt(a, n)
# This code is contributed by splevel62.
Output1 2
1 3
2 1
2 3
3 1
3 2
In this example, it is evident that the print statement gets executed n2 times. Now linear functions g(n), logarithmic functions g(log n), constant functions g(1) will always grow at a lesser rate than n2 when the input range tends to infinity therefore, the best-case running time of this program can be Ω(log n), Ω(n), Ω(1), or any function g(n) which is less than n2 when n tends to infinity.
When to use Big-Omega Ω notation?
Big-Omega Ω notation is the least used notation for the analysis of algorithms because it can make a correct but imprecise statement over the performance of an algorithm.
Suppose a person takes 100 minutes to complete a task, then using Ω notation it can be stated that the person takes more than 10 minutes to do the task, this statement is correct but not precise as it doesn’t mention the upper bound of the time taken. Similarly, using Ω notation we can say that the best-case running time for the binary search is Ω(1), which is true because we know that binary search would at least take constant time to execute but not very precise as in most of the cases binary search takes log(n) operations to complete.
Difference between Big-Omega Ω and Little-Omega ω notation:
Parameters
| Big-Omega Ω Notation
| Little-Omega ω Notation
|
---|
Description
| Big-Omega (Ω) describes the tight lower bound notation.
| Little-Omega(ω) describes the loose lower bound notation.
|
---|
Formal Definition
| Given two functions g(n) and f(n), we say that f(n) = Ω(g(n)), if there exists constants c > 0 and n0 >= 0 such that f(n) >= c*g(n) for all n >= n0.
| Given two functions g(n) and f(n), we say that f(n) = ω(g(n)), if there exists constants c > 0 and n0 >= 0 such that f(n) > c*g(n) for all n >= n0.
|
---|
Representation
| f(n) = ω(g(n)) represents that f(n) grows strictly faster than g(n) asymptotically.
| f(n) = Ω(g(n)) represents that f(n) grows at least as fast as g(n) asymptotically.
|
---|
Frequently Asked Questions about Big-Omega Ω notation:
Question 1: What is Big-Omega Ω notation?
Answer: Big-Omega Ω notation, is a way to express the asymptotic lower bound of an algorithm’s time complexity, since it analyses the best-case situation of algorithm. It provides a lower limit on the time taken by an algorithm in terms of the size of the input.
Question 2: What is the equation of Big-Omega (Ω)?
Answer: The equation for Big-Omega Ω is:
Given two functions g(n) and f(n), we say that f(n) = Ω(g(n)), if there exists constants c > 0 and n0 >= 0 such that f(n) >= c*g(n) for all n >= n0.
Question 3: What does the notation Omega mean?
Answer: Big-Omega Ω means the asymptotic lower bound of a function. In other words, we use Big-Ω represents the least amount of time or space the algorithm takes to run.
Question 4: What is the difference between Big-Omega Ω and Little-Omega ω notation?
Answer: Big-Omega (Ω) describes the tight lower bound notation whereas Little-Omega(ω) describes the loose lower bound notation.
Question 5: Why is Big-Omega Ω used?
Answer: Big-Omega Ω is used to specify the best-case time complexity or the lower bound of a function. It is used when we want to know the least amount of time that a function will take to execute.
Question 6: How is Big Omega Ω notation different from Big O notation?
Answer: Big Omega notation (Ω(f(n))) represents the lower bound of an algorithm’s complexity, indicating that the algorithm will not perform better than this lower bound, Whereas Big O notation (O(f(n))) represents the upper bound or worst-case complexity of an algorithm.
Question 7: What does it mean if an algorithm has a Big Omega complexity of Ω(n)?
Answer: If an algorithm has a Big Omega complexity of Ω(n), it means that the algorithm’s performance is at least linear in relation to the input size. In other words, the algorithm’s running time or space usage grows at least proportionally to the input size.
Question 8: Can an algorithm have multiple Big Omega Ω complexities?
Answer: Yes, an algorithm can have multiple Big Omega complexities depending on different input scenarios or conditions within the algorithm. Each complexity represents a lower bound for specific cases.
Question 9: How does Big Omega complexity relate to best-case performance analysis?
Answer: Big Omega complexity is closely related to best-case performance analysis because it represents the lower bound of an algorithm’s performance. However, it’s important to note that the best-case scenario may not always coincide with the Big Omega complexity.
Question 10: In what scenarios is understanding Big Omega complexity particularly important?
Answer: Understanding Big Omega complexity is important when we need to guarantee a certain level of performance or when we want to compare the efficiencies of different algorithms in terms of their lower bounds.
Related Articles:
Similar Reads
Analysis of Algorithms
Analysis of Algorithms is a fundamental aspect of computer science that involves evaluating performance of algorithms and programs. Efficiency is measured in terms of time and space. Basics on Analysis of Algorithms:Why is Analysis Important?Order of GrowthAsymptotic Analysis Worst, Average and Best
1 min read
Complete Guide On Complexity Analysis - Data Structure and Algorithms Tutorial
Complexity analysis is defined as a technique to characterise the time taken by an algorithm with respect to input size (independent from the machine, language and compiler). It is used for evaluating the variations of execution time on different algorithms. What is the need for Complexity Analysis?
15+ min read
Why is Analysis of Algorithm important?
Why is Performance of Algorithms Important ? There are many important things that should be taken care of, like user-friendliness, modularity, security, maintainability, etc. Why worry about performance? The answer to this is simple, we can have all the above things only if we have performance. So p
2 min read
Types of Asymptotic Notations in Complexity Analysis of Algorithms
We have discussed Asymptotic Analysis, and Worst, Average, and Best Cases of Algorithms. The main idea of asymptotic analysis is to have a measure of the efficiency of algorithms that don't depend on machine-specific constants and don't require algorithms to be implemented and time taken by programs
8 min read
Worst, Average and Best Case Analysis of Algorithms
In the previous post, we discussed how Asymptotic analysis overcomes the problems of the naive way of analyzing algorithms. Now let us learn about What is Worst, Average, and Best cases of an algorithm: 1. Worst Case Analysis (Mostly used) In the worst-case analysis, we calculate the upper bound on
10 min read
Asymptotic Analysis
Given two algorithms for a task, how do we find out which one is better? One naive way of doing this is - to implement both the algorithms and run the two programs on your computer for different inputs and see which one takes less time. There are many problems with this approach for the analysis of
3 min read
How to Analyse Loops for Complexity Analysis of Algorithms
We have discussed Asymptotic Analysis, Worst, Average and Best Cases and Asymptotic Notations in previous posts. In this post, an analysis of iterative programs with simple examples is discussed. The analysis of loops for the complexity analysis of algorithms involves finding the number of operation
15+ min read
Sample Practice Problems on Complexity Analysis of Algorithms
Prerequisite: Asymptotic Analysis, Worst, Average and Best Cases, Asymptotic Notations, Analysis of loops. Problem 1: Find the complexity of the below recurrence: { 3T(n-1), if n>0,T(n) = { 1, otherwise Solution: Let us solve using substitution. T(n) = 3T(n-1) = 3(3T(n-2)) = 32T(n-2) = 33T(n-3) .
15 min read
Basics on Analysis of Algorithms
Why is Analysis of Algorithm important?
Why is Performance of Algorithms Important ? There are many important things that should be taken care of, like user-friendliness, modularity, security, maintainability, etc. Why worry about performance? The answer to this is simple, we can have all the above things only if we have performance. So p
2 min read
Asymptotic Analysis
Given two algorithms for a task, how do we find out which one is better? One naive way of doing this is - to implement both the algorithms and run the two programs on your computer for different inputs and see which one takes less time. There are many problems with this approach for the analysis of
3 min read
Worst, Average and Best Case Analysis of Algorithms
In the previous post, we discussed how Asymptotic analysis overcomes the problems of the naive way of analyzing algorithms. Now let us learn about What is Worst, Average, and Best cases of an algorithm: 1. Worst Case Analysis (Mostly used) In the worst-case analysis, we calculate the upper bound on
10 min read
Types of Asymptotic Notations in Complexity Analysis of Algorithms
We have discussed Asymptotic Analysis, and Worst, Average, and Best Cases of Algorithms. The main idea of asymptotic analysis is to have a measure of the efficiency of algorithms that don't depend on machine-specific constants and don't require algorithms to be implemented and time taken by programs
8 min read
How to Analyse Loops for Complexity Analysis of Algorithms
We have discussed Asymptotic Analysis, Worst, Average and Best Cases and Asymptotic Notations in previous posts. In this post, an analysis of iterative programs with simple examples is discussed. The analysis of loops for the complexity analysis of algorithms involves finding the number of operation
15+ min read
How to analyse Complexity of Recurrence Relation
The analysis of the complexity of a recurrence relation involves finding the asymptotic upper bound on the running time of a recursive algorithm. This is usually done by finding a closed-form expression for the number of operations performed by the algorithm as a function of the input size, and then
7 min read
Introduction to Amortized Analysis
Amortized Analysis is used for algorithms where an occasional operation is very slow, but most of the other operations are faster. In Amortized Analysis, we analyze a sequence of operations and guarantee a worst-case average time that is lower than the worst-case time of a particularly expensive ope
10 min read
Asymptotic Notations
Big O Notation Tutorial - A Guide to Big O Analysis
Big O notation is a powerful tool used in computer science to describe the time complexity or space complexity of algorithms. It provides a standardized way to compare the efficiency of different algorithms in terms of their worst-case performance. Understanding Big O notation is essential for analy
11 min read
Difference between Big O vs Big Theta Θ vs Big Omega Ω Notations
Prerequisite - Asymptotic Notations, Properties of Asymptotic Notations, Analysis of Algorithms1. Big O notation (O): It is defined as upper bound and upper bound on an algorithm is the most amount of time required ( the worst case performance).Big O notation is used to describe the asymptotic upper
4 min read
Examples of Big-O analysis
Prerequisite: Analysis of Algorithms | Big-O analysis In the previous article, the analysis of the algorithm using Big O asymptotic notation is discussed. In this article, some examples are discussed to illustrate the Big O time complexity notation and also learn how to compute the time complexity o
13 min read
Difference between big O notations and tilde
In asymptotic analysis of algorithms we often encounter terms like Big-Oh, Omega, Theta and Tilde, which describe the performance of an algorithm. You can refer to the following links to get more insights about asymptotic analysis : Analysis of Algorithms Different NotationsDifference between Big Oh
4 min read
Analysis of Algorithms | Big-Omega Ω Notation
In the analysis of algorithms, asymptotic notations are used to evaluate the performance of an algorithm, in its best cases and worst cases. This article will discuss Big-Omega Notation represented by a Greek letter (Ω). Table of Content What is Big-Omega Ω Notation?Definition of Big-Omega Ω Notatio
9 min read
Analysis of Algorithms | Θ (Theta) Notation
In the analysis of algorithms, asymptotic notations are used to evaluate the performance of an algorithm by providing an exact order of growth. This article will discuss Big - Theta notations represented by a Greek letter (Θ). Definition: Let g and f be the function from the set of natural numbers t
6 min read
Some Advance Topics
P, NP, CoNP, NP hard and NP complete | Complexity Classes
In computer science, there exist some problems whose solutions are not yet found, the problems are divided into classes known as Complexity Classes. In complexity theory, a Complexity Class is a set of problems with related complexity. These classes help scientists to group problems based on how muc
5 min read
Can Run Time Complexity of a comparison-based sorting algorithm be less than N logN?
Sorting algorithms are the means to sort a given set of data in an order according to the requirement of the user. They are primarily used to sort data in an increasing or decreasing manner. There are two types of sorting algorithms: Comparison-based sorting algorithmsNon-comparison-based sorting al
3 min read
Why does accessing an Array element take O(1) time?
An array is a linear data structure. In an array, the operation to fetch a value takes constant time i.e., O(1). Let us see why is it so. Why the complexity of fetching value is O(1)? As Arrays are allocated contiguously in memory, Fetching a value via an index of the array is an arithmetic operatio
2 min read
What is the time efficiency of the push(), pop(), isEmpty() and peek() operations of Stacks?
Stack is a linear data structure that follows the LIFO (last in first out) order i.e., the data are entered from one end and while they are removed, they will be removed from the same end. The few most performed operations in a stack are: push()pop()isEmpty()peek()Now let us check the time efficienc
2 min read
Complexity Proofs
Proof that Clique Decision problem is NP-Complete
Prerequisite: NP-Completeness A clique is a subgraph of a graph such that all the vertices in this subgraph are connected with each other that is the subgraph is a complete graph. The Maximal Clique Problem is to find the maximum sized clique of a given graph G, that is a complete graph which is a s
4 min read
Proof that Independent Set in Graph theory is NP Complete
Prerequisite: NP-Completeness, Independent set. An Independent Set S of graph G = (V, E) is a set of vertices such that no two vertices in S are adjacent to each other. It consists of non- adjacent vertices. Problem: Given a graph G(V, E) and an integer k, the problem is to determine if the graph co
5 min read
Prove that a problem consisting of Clique and Independent Set is NP Complete
Prerequisite: NP-Completeness, NP Class, Clique, Independent Set Problem: Given an undirected graph G = (V, E) and an integer K, determine if a clique of size K as well as an independent set (IS) of size K, exists. Demonstrate that it is an NP Complete. Explanation: A Clique is a subgraph of a graph
6 min read
Prove that Dense Subgraph is NP Complete by Generalisation
Prerequisites: NP-Completeness, NP Class, Dense Subgraph Problem: Given graph G = (V, E) and two integers a and b. A set of a number of vertices of G such that there are at least b edges between them is known as the Dense Subgraph of graph G. Explanation: To prove the Dense Subgraph problem as NP-c
3 min read
Prove that Sparse Graph is NP-Complete
Prerequisite: NP-Completeness, NP Class, Sparse Graph, Independent Set Problem: Given graph G = (V, E) and two integers a and b. A set of a number of vertices of G such that there are at most b edges between them is known as the Sparse Subgraph of graph G. Explanation: Sparse Subgraph problem is def
4 min read
Top MCQs on Complexity Analysis of Algorithms with Answers
Complexity analysis is defined as a technique to characterise the time taken by an algorithm with respect to input size (independent from the machine, language and compiler). It is used for evaluating the variations of execution time on different algorithms. More on Complexity Analysis [mtouchquiz 1
1 min read